February 8, 2025
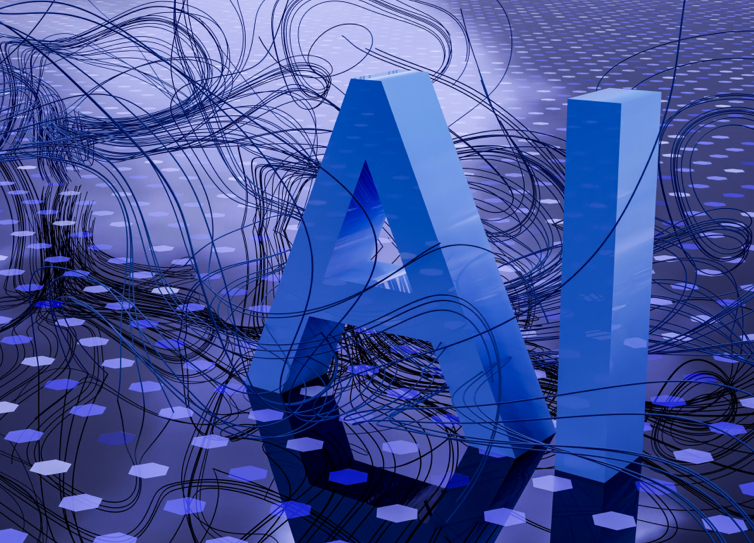
Are automated debtor credit limits sufficiently reliable for use in trade credit insurance and trade finance environments?
We hear a lot about “AI” and “Turning Data into Knowledge.” However, do these advances realistically have a place in debtor credit limit management and should insurers or funders rely on them?
From our experience, I’d say the answer is a qualified yes. Data, of course, is key, and a seller’s trading experience in isolation isn’t enough to set reliable debtor credit limits. After all, trading experience alone doesn’t tell you if a debtor is or becomes insolvent and invoice data doesn’t tell you what a debtor does or how long it’s been in business.
We’ve found that a tranched approach often produces the most reliable results; automatically set smaller debtor credit limits using a combination of seller trading experience and basic firmographic data such as debtor activity, age and current operating status. For higher value credit limits, enhance the data with risk scores and probability of default, then for the largest or most challenging credit limits, add a manually review capability.
Some of our insurance partners issue non-cancellable debtor credit limits, which become more difficult to manage when based on a seller’s invoice data. Live trading experience is great for giving early warning signs of changing payment patterns, but how derogatory must that experience become before a debtor credit limit can be cancelled?
Across our trade credit insurance and trade finance products we are setting hundreds of thousands of automated global debtor credit limits, predominantly using a combination of credit agency firmographic data and the payment experience of one or more sellers trading with the subject debtor. They allow credit limit decisions to be made on every debtor in a portfolio-wide insurance or funding program and can remove the uncertainty often associated with discretionary cover.
But to maintain acceptable loss ratios, automated limits need to be used conditionally and in combination with the backing of a manual credit risk analysis capability.
Ready to learn more?
Contact us to find out more, and talk to a member of the Aronova team.
Subscribe for premium content
To continue reading premium content like this and to receive future notifications about articles from Aronova please sign up to receive our marketing communications.
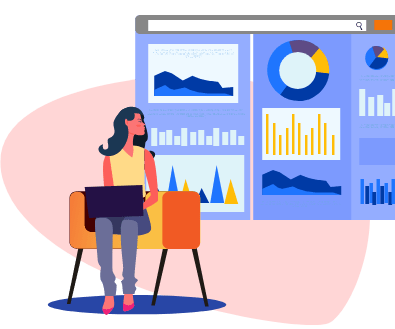
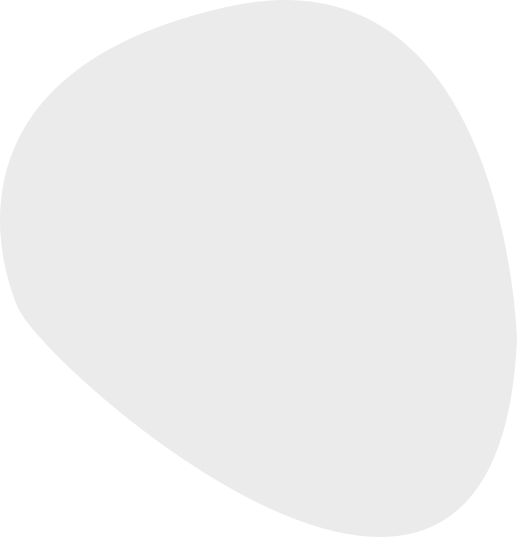